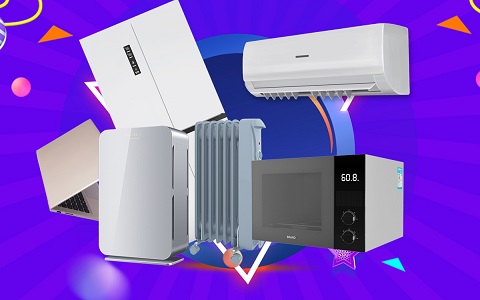
(资料图)
2023美国大学生数学建模竞赛思路分析
资料思路分享Q群:714452621
1 算法原理与流程
CBA算法作为分类算法,他的分类情况也就是给定一些预先知道的属性,然后叫你判断出他的决策属性是哪个值。判断的依据就是Apriori算法挖掘出的频繁项,如果一个项集中包含预先知道的属性,同时也包含分类属性值,然后我们计算此频繁项能否导出已知属性值推出决策属性值的关联规则,如果满足规则的最小置信度的要求,那么可以把频繁项中的决策属性值作为最后的分类结果。具体的算法细节如下:
1、输入数据记录,就是一条条的属性值。
2、对属性值做数字的替换(按照列从上往下寻找属性值),就类似于Apriori中的一条条事务记录。
3、根据这个转化后的事务记录,进行Apriori算法计算,挖掘出频繁项集。
4、输入查询的属性值,找出符合条件的频繁项集(需要包含查询属性和分类决策属性),如果能够推导出这样的关联规则,就算分类成功,输出分类结果。
算法实现
这里给出CBA关联分析的实现类:
package DataMining_CBA; import java.io.BufferedReader;import java.io.File;import java.io.FileReader;import java.io.IOException;import java.util.ArrayList;import java.util.HashMap;import java.util.regex.Matcher;import java.util.regex.Pattern; import DataMining_CBA.AprioriTool.AprioriTool;import DataMining_CBA.AprioriTool.FrequentItem; /** * CBA算法(关联规则分类)工具类 * * @author lyq * */public class CBATool { // 年龄的类别划分 public final String AGE = "Age"; public final String AGE_YOUNG = "Young"; public final String AGE_MIDDLE_AGED = "Middle_aged"; public final String AGE_Senior = "Senior"; // 测试数据地址 private String filePath; // 最小支持度阈值率 private double minSupportRate; // 最小置信度阈值,用来判断是否能够成为关联规则 private double minConf; // 最小支持度 private int minSupportCount; // 属性列名称 private String[] attrNames; // 类别属性所代表的数字集合 private ArrayList<Integer> classTypes; // 用二维数组保存测试数据 private ArrayList<String[]> totalDatas; // Apriori算法工具类 private AprioriTool aprioriTool; // 属性到数字的映射图 private HashMap<String, Integer> attr2Num; private HashMap<Integer, String> num2Attr; public CBATool(String filePath, double minSupportRate, double minConf) { this.filePath = filePath; this.minConf = minConf; this.minSupportRate = minSupportRate; readDataFile(); } /** * 从文件中读取数据 */ private void readDataFile() { File file = new File(filePath); ArrayList<String[]> dataArray = new ArrayList<String[]>(); try { BufferedReader in = new BufferedReader(new FileReader(file)); String str; String[] tempArray; while ((str = in.readLine()) != null) { tempArray = str.split(" "); dataArray.add(tempArray); } in.close(); } catch (IOException e) { e.getStackTrace(); } totalDatas = new ArrayList<>(); for (String[] array : dataArray) { totalDatas.add(array); } attrNames = totalDatas.get(0); minSupportCount = (int) (minSupportRate * totalDatas.size()); attributeReplace(); } /** * 属性值的替换,替换成数字的形式,以便进行频繁项的挖掘 */ private void attributeReplace() { int currentValue = 1; int num = 0; String s; // 属性名到数字的映射图 attr2Num = new HashMap<>(); num2Attr = new HashMap<>(); classTypes = new ArrayList<>(); // 按照1列列的方式来,从左往右边扫描,跳过列名称行和id列 for (int j = 1; j < attrNames.length; j++) { for (int i = 1; i < totalDatas.size(); i++) { s = totalDatas.get(i)[j]; // 如果是数字形式的,这里只做年龄类别转换,其他的数字情况类似 if (attrNames[j].equals(AGE)) { num = Integer.parseInt(s); if (num <= 20 && num > 0) { totalDatas.get(i)[j] = AGE_YOUNG; } else if (num > 20 && num <= 40) { totalDatas.get(i)[j] = AGE_MIDDLE_AGED; } else if (num > 40) { totalDatas.get(i)[j] = AGE_Senior; } } if (!attr2Num.containsKey(totalDatas.get(i)[j])) { attr2Num.put(totalDatas.get(i)[j], currentValue); num2Attr.put(currentValue, totalDatas.get(i)[j]); if (j == attrNames.length - 1) { // 如果是组后一列,说明是分类类别列,记录下来 classTypes.add(currentValue); } currentValue++; } } } // 对原始的数据作属性替换,每条记录变为类似于事务数据的形式 for (int i = 1; i < totalDatas.size(); i++) { for (int j = 1; j < attrNames.length; j++) { s = totalDatas.get(i)[j]; if (attr2Num.containsKey(s)) { totalDatas.get(i)[j] = attr2Num.get(s) + ""; } } } } /** * Apriori计算全部频繁项集 * @return */ private ArrayList<FrequentItem> aprioriCalculate() { String[] tempArray; ArrayList<FrequentItem> totalFrequentItems; ArrayList<String[]> copyData = (ArrayList<String[]>) totalDatas.clone(); // 去除属性名称行 copyData.remove(0); // 去除首列ID for (int i = 0; i < copyData.size(); i++) { String[] array = copyData.get(i); tempArray = new String[array.length - 1]; System.arraycopy(array, 1, tempArray, 0, tempArray.length); copyData.set(i, tempArray); } aprioriTool = new AprioriTool(copyData, minSupportCount); aprioriTool.computeLink(); totalFrequentItems = aprioriTool.getTotalFrequentItems(); return totalFrequentItems; } /** * 基于关联规则的分类 * * @param attrValues * 预先知道的一些属性 * @return */ public String CBAJudge(String attrValues) { int value = 0; // 最终分类类别 String classType = null; String[] tempArray; // 已知的属性值 ArrayList<String> attrValueList = new ArrayList<>(); ArrayList<FrequentItem> totalFrequentItems; totalFrequentItems = aprioriCalculate(); // 将查询条件进行逐一属性的分割 String[] array = attrValues.split(","); for (String record : array) { tempArray = record.split("="); value = attr2Num.get(tempArray[1]); attrValueList.add(value + ""); } // 在频繁项集中寻找符合条件的项 for (FrequentItem item : totalFrequentItems) { // 过滤掉不满足个数频繁项 if (item.getIdArray().length < (attrValueList.size() + 1)) { continue; } // 要保证查询的属性都包含在频繁项集中 if (itemIsSatisfied(item, attrValueList)) { tempArray = item.getIdArray(); classType = classificationBaseRules(tempArray); if (classType != null) { // 作属性替换 classType = num2Attr.get(Integer.parseInt(classType)); break; } } } return classType; } /** * 基于关联规则进行分类 * * @param items * 频繁项 * @return */ private String classificationBaseRules(String[] items) { String classType = null; String[] arrayTemp; int count1 = 0; int count2 = 0; // 置信度 double confidenceRate; String[] noClassTypeItems = new String[items.length - 1]; for (int i = 0, k = 0; i < items.length; i++) { if (!classTypes.contains(Integer.parseInt(items[i]))) { noClassTypeItems[k] = items[i]; k++; } else { classType = items[i]; } } for (String[] array : totalDatas) { // 去除ID数字号 arrayTemp = new String[array.length - 1]; System.arraycopy(array, 1, arrayTemp, 0, array.length - 1); if (isStrArrayContain(arrayTemp, noClassTypeItems)) { count1++; if (isStrArrayContain(arrayTemp, items)) { count2++; } } } // 做置信度的计算 confidenceRate = count1 * 1.0 / count2; if (confidenceRate >= minConf) { return classType; } else { // 如果不满足最小置信度要求,则此关联规则无效 return null; } } /** * 判断单个字符是否包含在字符数组中 * * @param array * 字符数组 * @param s * 判断的单字符 * @return */ private boolean strIsContained(String[] array, String s) { boolean isContained = false; for (String str : array) { if (str.equals(s)) { isContained = true; break; } } return isContained; } /** * 数组array2是否包含于array1中,不需要完全一样 * * @param array1 * @param array2 * @return */ private boolean isStrArrayContain(String[] array1, String[] array2) { boolean isContain = true; for (String s2 : array2) { isContain = false; for (String s1 : array1) { // 只要s2字符存在于array1中,这个字符就算包含在array1中 if (s2.equals(s1)) { isContain = true; break; } } // 一旦发现不包含的字符,则array2数组不包含于array1中 if (!isContain) { break; } } return isContain; } /** * 判断频繁项集是否满足查询 * * @param item * 待判断的频繁项集 * @param attrValues * 查询的属性值列表 * @return */ private boolean itemIsSatisfied(FrequentItem item, ArrayList<String> attrValues) { boolean isContained = false; String[] array = item.getIdArray(); for (String s : attrValues) { isContained = true; if (!strIsContained(array, s)) { isContained = false; break; } if (!isContained) { break; } } if (isContained) { isContained = false; // 还要验证是否频繁项集中是否包含分类属性 for (Integer type : classTypes) { if (strIsContained(array, type + "")) { isContained = true; break; } } } return isContained; } }
2023美国大学生数学建模竞赛思路分析
资料思路分享Q群:714452621